Data Analytics and Predictions
Data analytics is essential for extracting meaningful insights from raw data, enabling evidence-based decision-making and increased efficiency. Predictive analytics, a vital subset, uses historical data and advanced modeling to forecast future trends. This technology is widely applied across industries, offering benefits such as improved security, risk reduction, and operational efficiency.
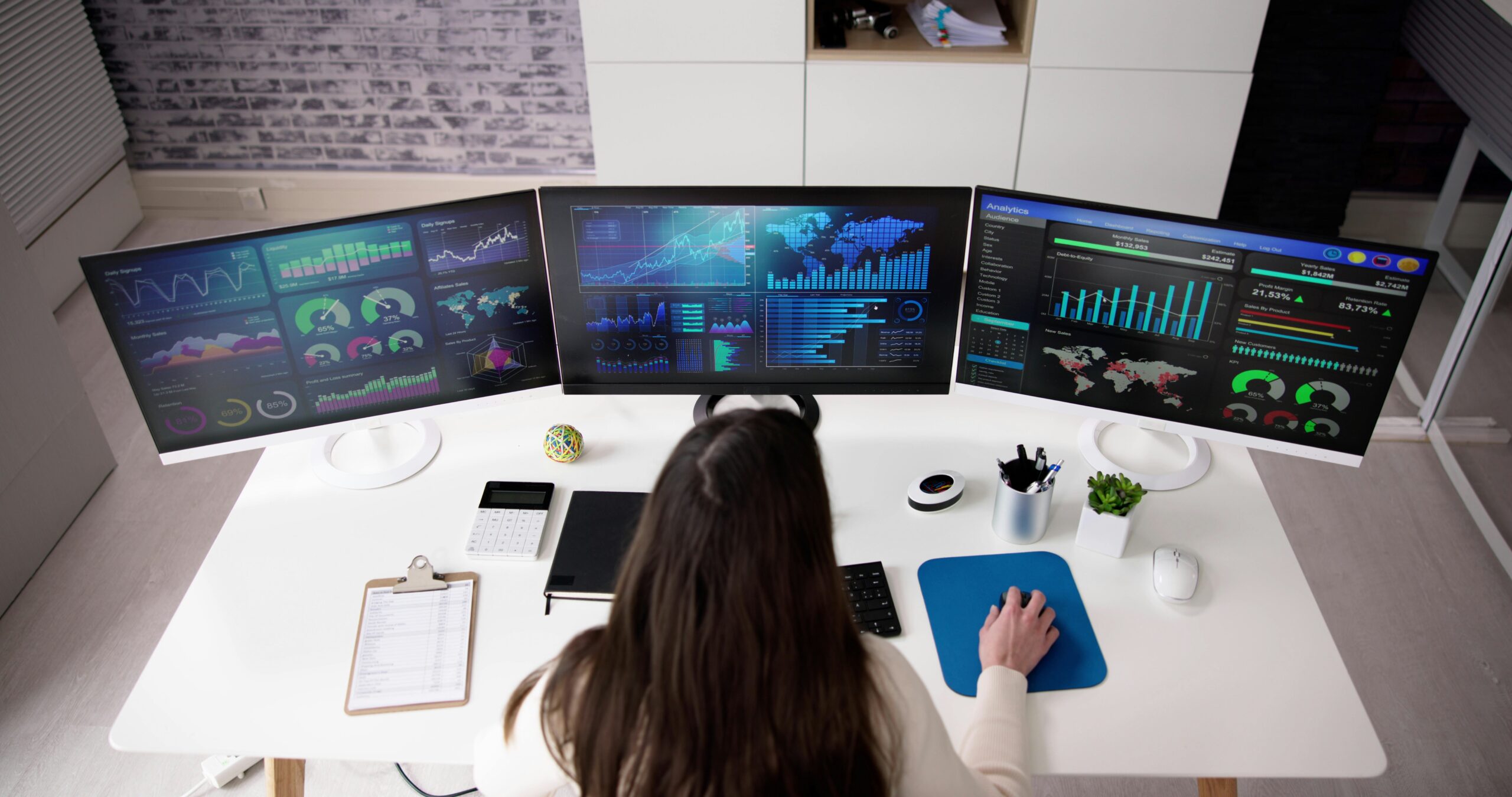
Understanding Data Analytics
Data analytics involves examining raw data to identify trends and answer questions. It encompasses various methods and objectives that vary by industry. The process starts with descriptive analytics, which summarizes historical data, and progresses to advanced analytics, employing techniques like machine learning to forecast future trends. Enhanced by modern tools and vast data sets, data analytics allows businesses to derive significant insights from complex data.
The Necessity of Data Analytics
Data analytics is crucial for organizing and analyzing raw data to provide actionable insights. It enables businesses to formulate evidence-based strategies, understand customer behavior, and enhance productivity. Organizations that leverage data analytics can swiftly adapt to changes, boosting revenue, reducing costs, and fostering innovation. In the digital age, data-driven decision-making is vital for effective leadership across all sectors.
Connecting Data Analytics and Predictive Analytics
Predictive analytics, a subset of advanced analytics, uses historical data with statistical models and machine learning to forecast future outcomes. It helps organizations identify risks and opportunities by recognizing patterns in large datasets. Techniques such as regression models and neural networks allow companies to make informed predictions about future events, integrating insights from vast and diverse data sources.
Various Predictive Modeling Techniques
Predictive models analyze historical data to detect patterns and predict future trends. Common techniques include classification, clustering, and time series models. Each method offers unique benefits for understanding data and making predictions, helping organizations optimize their strategies and operations.
Practical Applications of Predictive Analytics
Banking: In the banking sector, predictive analytics utilizes machine learning and quantitative methods to forecast customer behaviors and identify potential risks. For instance, banks can determine which clients are likely to default on loans, assess customer risk profiles, and pinpoint the most profitable customers for targeted marketing efforts. Predictive models can also identify fraudulent transactions by recognizing unusual spending patterns. By applying these insights, banks can allocate their resources more effectively and mitigate financial risks.
Healthcare: Predictive analytics in healthcare plays a crucial role in managing chronic illnesses and tracking infections like sepsis. Geisinger Health has employed predictive models to analyze health records of over 10,000 sepsis patients. This model has significantly improved the accuracy of sepsis diagnoses and treatment plans, leading to higher survival rates. Predictive analytics helps healthcare providers anticipate patient needs, streamline treatment plans, and improve overall patient outcomes by leveraging vast amounts of health data.
Marketing and Sales: Marketing and sales teams benefit from predictive analytics by adopting a proactive approach to customer engagement. Predictive models can identify customers likely to churn, allowing sales teams to intervene early and improve retention rates. Additionally, marketing teams use predictive data to develop cross-sell strategies, often through recommendation engines on websites. This data-driven approach enables companies to personalize marketing efforts, increase customer loyalty, and optimize sales processes throughout the customer lifecycle.
Supply Chain: In supply chain management, predictive analytics helps businesses maintain optimal inventory levels and develop effective pricing strategies. By analyzing past shipping data and market trends, companies like FleetPride can better predict demand and set supply thresholds, avoiding overstocking and minimizing costs. Predictive models also allow companies to assess the long-term financial impact of changes in import costs and pricing strategies, ensuring that supply meets demand efficiently and profitably. This predictive capability enables businesses to stay competitive and responsive to market changes.
Advantages of Predictive Analytics
Predictive analytics provides businesses with a competitive edge by forecasting future trends based on past data. It enhances security through anomaly detection, reduces risks by identifying potential defaults or inadequate coverage, improves operational efficiency by predicting maintenance needs, and aids in strategic decision-making by offering insights into potential outcomes.